Our Data Science Courses
➤ Data Science for Finance Professionals
➤ Data Science for Healthcare Professionals
➤ Data Science with International Supply Chain
➤ Data Science with Digital Transformation
➤ Data Science - 3 Months
➤ Data Science - 6 Months
➤ Data Science with NLP
➤ Certified Data Analyst professional with Tableau
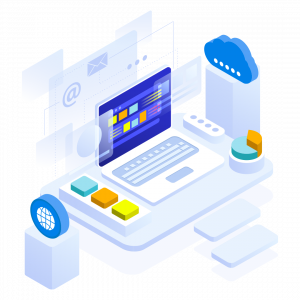
Who We Are?
We are pioneers of a new and powerful era of education. At Chools, we strive to bring you the best and make you employable.
With carefully curated content and 24×7 support at your fingertips, you will never have to look elsewhere again.
We are the best trainers in the latest, coveted technologies across the globe, and we can help you carve your career. Come learn with us and give yourself the gift of knowledge.
What We Do?
We have crafted a unique pedagogy model to let learning be fun, self-paced, and instructor-led.
We are devoted to making quality education affordable with personalized guidance, lifetime course access, 24×7 support, live projects, and resume and interview preparation.
Our expert instructors aim to deliver real-time technical experience and keep pushing their boundaries to ensure everything is relevant.
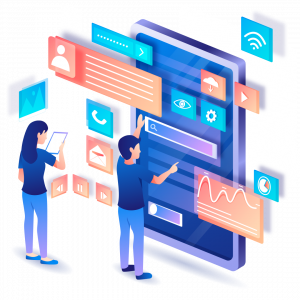
High Paying Skills for Data Scientists
Data scientists are highly paid because their skills are integral to driving business success, innovation, and competitive advantage in the data-driven world. The demand for their expertise, combined with their ability to translate data into actionable insights, makes them invaluable assets to organizations.
Here are some key factors contributing to why data scientists are often paid significant salaries:
● Specialized Skills: Data scientists possess a unique skill set that includes expertise in statistics, programming, machine learning, data analysis, and domain knowledge. These specialized skills are in high demand as organizations seek to harness the power of data for insights and decision-making.
● Data-Driven Decision-Making: In today’s data-driven business landscape, organizations rely on data scientists to analyze vast amounts of data and extract meaningful insights. Data-driven decisions can lead to increased efficiency, cost savings, and competitive advantages, justifying higher salaries.
● Shortage of Talent: There is a shortage of skilled data scientists in the job market relative to the growing demand. This supply-demand gap drives up the salaries for experienced and qualified data scientists.
● Impact on Revenue: Effective data analysis can directly impact a company’s bottom line. Data scientists can uncover patterns, trends, and correlations that lead to better marketing strategies, improved products, optimized operations, and increased revenue.
● Complex Problem Solving: Data scientists are often tasked with solving complex and critical business problems. Their ability to design and implement intricate machine learning models, algorithms, and data pipelines adds substantial value to organizations.
● Cross-Functional Collaboration: Data scientists collaborate with various departments such as marketing, finance, operations, and research. Their insights influence multiple facets of a business, making them valuable assets.
● Innovation and Research: Data scientists contribute to innovation by developing new algorithms, techniques, and methodologies. Their research can lead to breakthroughs in AI, machine learning, and other fields, driving up their value and compensation.
● High ROI: Companies recognize the return on investment (ROI) of hiring skilled data scientists. The insights and solutions provided by data scientists often result in substantial financial gains that far outweigh their salaries.
● Competitive Landscape: As organizations compete to attract and retain top talent, offering competitive salaries to data scientists is a way to stand out and secure skilled professionals.
● Global Impact: Data scientists play a role in addressing global challenges such as healthcare, climate change, and social issues. Their work can have a positive impact on society, making their skills even more valuable.
● Continuous Learning: Data science is a rapidly evolving field that requires continuous learning to stay up-to-date with the latest tools, techniques, and trends. This commitment to ongoing education justifies higher compensation.
● Risk Mitigation: Data breaches and cyber threats are significant concerns for businesses. Data scientists contribute to risk mitigation by identifying vulnerabilities and developing security measures, further justifying their compensation.
Data Science Is A Hub of Job Junctions…
Data science is indeed a hub of vertical job opportunities. The field of data science encompasses a wide range of specializations and roles, each focusing on different aspects of data analysis, interpretation, and application.
Here are some of the vertical job opportunities within the data science field:
● Data Analyst: Data analysts focus on collecting, cleaning, and analyzing data to derive insights and make data-driven decisions. They often work with structured data and use tools like Excel, SQL, and visualization libraries to present findings.
● Data Scientist: Data scientists build predictive and prescriptive models using statistical techniques and machine learning algorithms. They uncover patterns and trends in data to solve complex business problems.
● Machine Learning Engineer: Machine learning engineers develop and deploy machine learning models at scale. They work on building and optimizing algorithms, feature engineering, and integrating models into production systems.
● Artificial Intelligence (AI) Engineer: AI engineers specialize in designing and implementing AI solutions, including natural language processing, computer vision, and robotics.
● Big Data Engineer: Big data engineers focus on designing, building, and maintaining large-scale data processing systems. They work with technologies like Hadoop, Spark, and distributed computing frameworks.
● Data Engineer: Data engineers are responsible for designing and maintaining data pipelines, data warehouses, and ETL (Extract, Transform, Load) processes to ensure data availability and quality.
● Business Intelligence Analyst: BI analysts create visualizations and reports to provide insights into business performance. They use tools like Tableau, Power BI, and QlikView.
● Quantitative Analyst (Quant): Quants apply statistical and mathematical models to financial and risk management problems. They often work in the finance industry to analyze market trends and develop trading strategies.
● Operations Analyst: Operations analysts use data to optimize business processes, improve efficiency, and reduce costs in various industries.
● Healthcare Data Analyst: Healthcare data analysts focus on analyzing medical and patient data to improve patient outcomes, optimize healthcare operations, and support medical research.
● Marketing Analyst: Marketing analysts use data to assess marketing campaigns, customer behavior, and market trends to inform marketing strategies.
● Sports Analyst: Sports analysts analyze player performance, game statistics, and team strategies to provide insights for coaches, players, and team management.
● Environmental Data Scientist: Environmental data scientists analyze data related to environmental factors, climate change, and sustainability to inform policy decisions and conservation efforts.
● Social Media Analyst: Social media analysts study social media data to understand consumer sentiment, engagement patterns, and marketing opportunities.
● Research Scientist: Research scientists focus on advancing the field of data science through academic research, contributing to new algorithms, methodologies, and theories.
● As data becomes more integral to decision-making across industries, the demand for data science expertise continues to grow, leading to even more specialized roles. It’s important to identify the niche that aligns with your interests, skills, and career goals.
Align Your Career With Data Science Certification
Choosing a certification that aligns with your career goals and interests is essential. Additionally, staying up-to-date with the latest trends and technologies in the IT industry is crucial for long-term success. these certifications can enhance your earning potential but require dedication and effort to achieve.
Here are some high-paying IT certifications:
● Certified Information Systems Security Professional (CISSP): This is a globally recognized certification in the field of information security. CISSP-certified professionals are sought after for their expertise in designing, implementing, and managing security programs to protect organizations from cyber threats.
● Certified Cloud Security Professional (CCSP): With the growing popularity of cloud computing, CCSP certification is highly valued. It focuses on cloud security architecture, design, operations, and compliance.
● Certified Information Systems Auditor (CISA): CISA-certified professionals specialize in auditing, control, and assurance of information systems and technology. They ensure that an organization’s IT systems are secure and provide accurate and reliable information.
● Certified in Risk and Information Systems Control (CRISC): CRISC certification is geared toward IT professionals who identify and manage IT and business risks. It’s particularly valuable for those involved in risk management and control assurance.
● Project Management Professional (PMP): While not exclusively an IT certification, PMP is highly regarded in the IT industry. It certifies your project management skills, which are essential in many IT projects.
● Cisco Certified Network Professional (CCNP): This certification validates advanced knowledge of networking. CCNP-certified professionals are skilled in planning, implementing, and troubleshooting both local and wide-area networks.
● Certified Data Professional (CDP): CDP certification is for data management professionals, including data analysts, data architects, and data engineers. It demonstrates expertise in data management and analytics.
● AWS Certified Solutions Architect – Professional: Amazon Web Services (AWS) certifications are in high demand due to the prevalence of cloud computing. The Solutions Architect – Professional certification demonstrates advanced AWS cloud architecture skills.
● Certified Ethical Hacker (CEH): CEH-certified professionals are trained to think and act like malicious hackers (ethical hacking) to identify vulnerabilities in systems. They play a critical role in securing systems and networks.
● Microsoft Certified: Azure Solutions Architect Expert: Similar to the AWS certification, this Microsoft Azure certification focuses on designing and implementing solutions in the Azure cloud environment.
Our Data Science Advisory Panel
What students like most about Chools
We asked hundreds of students what made their experience with Chools worthwhile and cherishable; here’s what they answered:
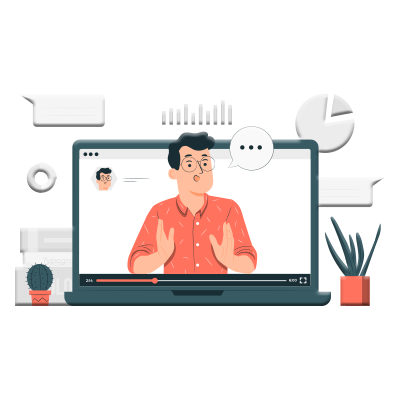
The Instructor
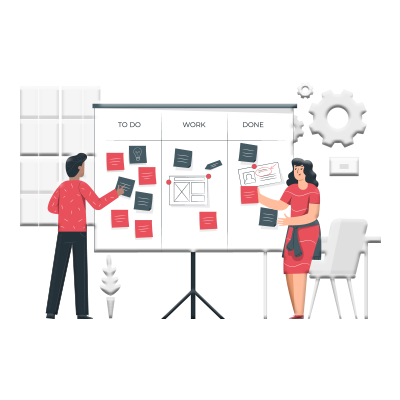
The Projects
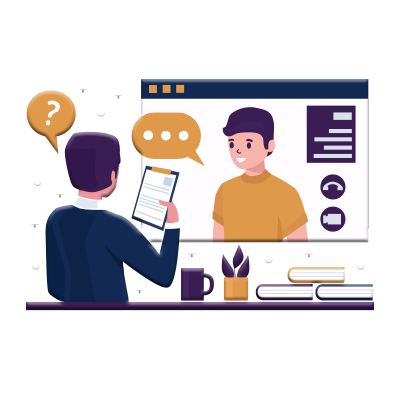
Mock Interviews
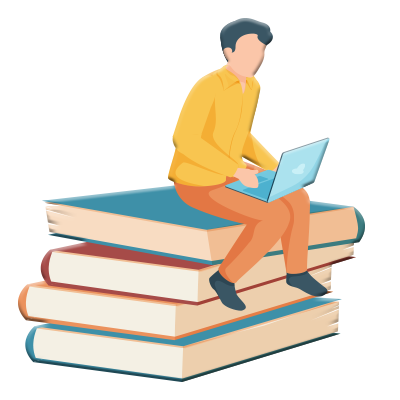
The Course Material
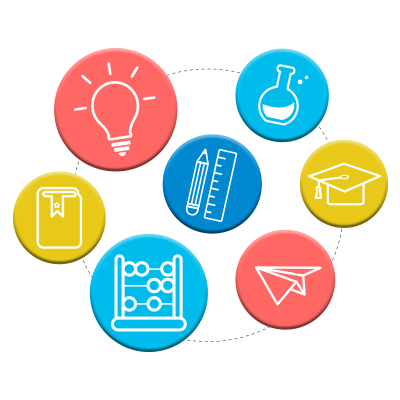
The Curriculum
Our Data Science Faculties
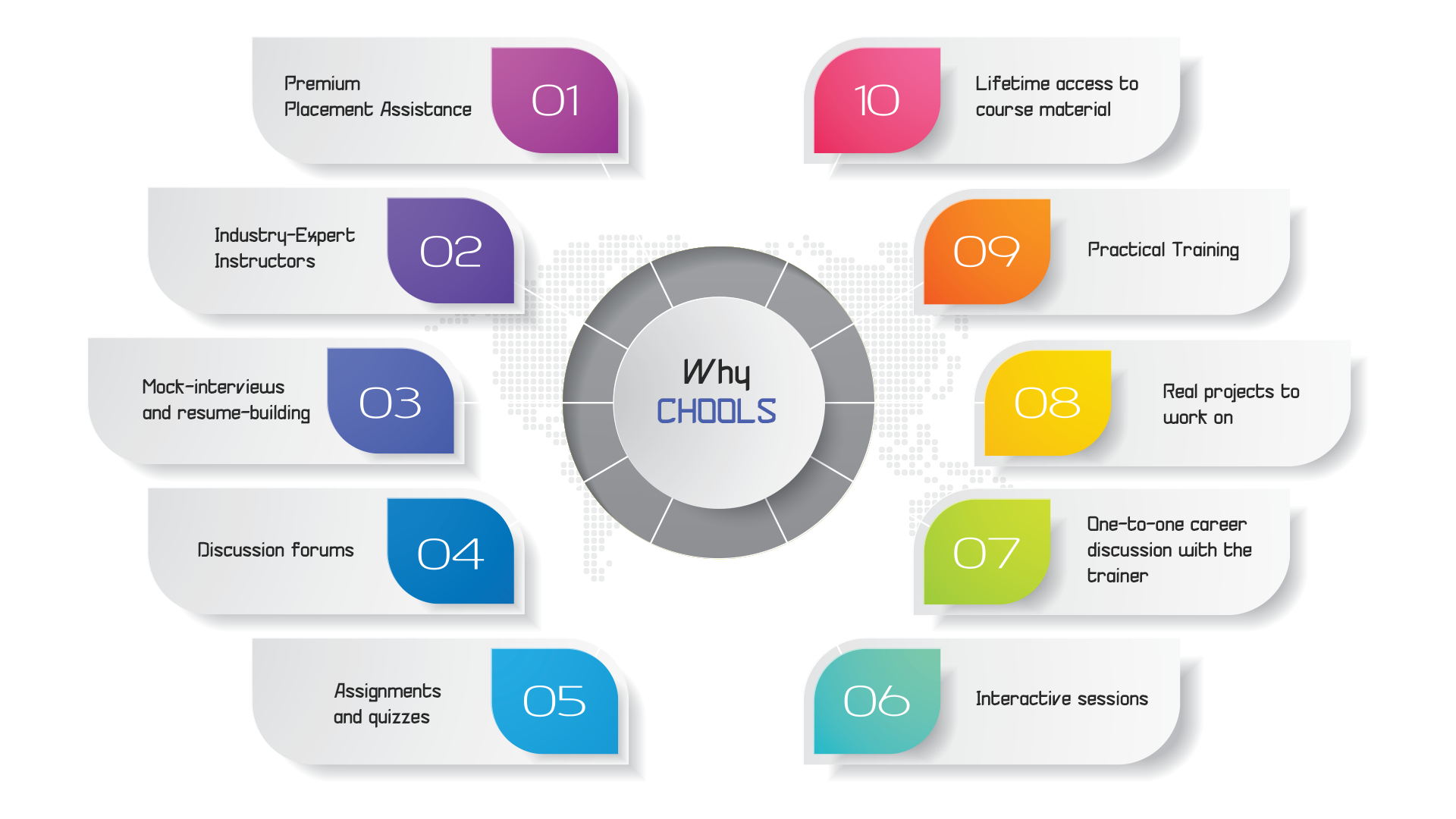
Take your first step towards RESULT.
Frequently Asked Questions about Data Science in 2023
Are Data Science Jobs in Demand?
With the fast development of modern technologies, data science is currently in extremely high demand, and this is only going to grow. For verification, just type “data science jobs” into Google or search for them in any job hunting website such as LinkedIn, Glassdoor, or Indeed. You will be overwhelmed by the number of job opportunities in this sphere.
There are many explanations for such a popularity. The amount of data produced around the world is rapidly accumulating every day, and every business requires data analyzing and predictive modeling to remain alive and successful in today’s highly competitive market. Scientific research in any field can be conducted only if enough historical data is collected. In other words, the more data an organization or science has gathered, the more reliable data-derived forecasts it can make.
That said, as with any other sphere, there were (and are) various “fashionable” trends in data science in different periods of its existence: machine learning, deep learning, data engineering, big data, and even Covid-19 data science.
What Does a Data Science Job Usually Entail?
Broadly speaking, data scientists gather and investigate the data relevant to a certain business or scientific task, and extract from it meaningful insights and hidden trends. Using machine learning and deep learning algorithms to build predictive models, they then create reports of their findings and communicate their results to non-technical shareholders. In turn, shareholders can then make strategic, data-driven decisions to improve the business.
All these steps require data scientists to be multi-skilled professionals. In particular, they should have sufficient knowledge of coding tools, be familiar with the mathematical principles behind various machine learning algorithms, understand the nuances of the business domain of a particular sphere of interest, follow data ethics, and have excellent communication skills so as to clearly explain complex ideas to a non-technical audience.
The above is an integral description of a classical data scientist role. Since this profession is relatively new however, different companies can have their own understanding of what the role of a data scientist should include. For example, in some cases, data scientists are rather data analysts focused on the investigation of the historical and current data without predicting future scenarios. In other companies, data scientists are supposed to use graphical user interface (GUI) applications to build machine learning models, so they practically don’t need to write any scripts. Finally, sometimes data scientists are meant to be data engineers whose main tasks are converting raw data to a usable form and designing and maintaining data storage infrastructure.
How Much Do Data Scientists Make?
As with many other professions, the answer to this question strongly depends on the country where the company is located, i.e., on the standard of living. For example, according to PayScale, at the time of writing (February 2022), the average salary of a data scientist in the United States is $97,038 per year, while in India, where this profession is also very highly demanded, it amounts to ₹860,454 per year, which is equivalent to $11,521.
Another significant factor that influences the salary of a data scientist in any country is the level of their seniority. Taking the United States again as an example, a junior data scientist earns $76,213 per year while a senior data scientist – $129,446 per year, i.e., almost 2 times more (Indeed).
The salary of a data scientist also depends on the company profile (a small company or a multinational corporation), area of focus (business or academic environment), and type of contract (permanent or temporary).
You can check the average salary of a data scientist in your country and for your seniority level using specialized websites such as Indeed, PaySclae and SlaryExpert You could also research information on the average salaries of other data-related professions, such as data engineer, data analyst, and data journalist.
What Are the Prerequisites to Start Learning Data Science?
While it is true that for mathematicians, statisticians, and programmers, the process of learning data science could be smoother and quicker, it doesn’t necessarily mean that a career in data science is completely inaccessible to people with different qualifications. Indeed, there are plenty of inspiring stories of the success of people who have entered this sphere from completely unrelated professions, made fast progress, and are now happily employed.
However, it is not correct either to claim that there are no prerequisites at all for a person to start learning data science. To succeed in your studies, you will need to be fascinated by the data and what is hidden behind it, an exploratory mindset, a certain amount of creativity, and a high motivation to learn data science.
Do I Need a University Degree to Learn Data Science, or Can I Learn Online?
While there is nothing wrong with a university degree in data science, you have to keep in mind one important thing: time matters. If you have recently graduated from college and are deciding on your further education, then a solid, well-grounded university degree in data science could be a great choice. If you are a career-changer instead, you probably won’t want to spend at least two more years on your studies before being employed.
Fortunately, if you are from the second category of people, there is good news for you: you can learn data science on an online bootcamps at a sufficient level to be employed as a data scientist. Moreover, this approach gives you much more freedom to organize your learning process, manage your time, practice a lot, and accelerate your progress whenever you feel ready.
In the world of work, it doesn’t matter how much time it takes for you to learn data science or whether or not you have a world-class certificate. What a potential employer really wants to see in a tech-competent candidate is a set of proven skills (confirmed by a portfolio of projects) relevant to a job position of interest.
How Long Does it Take to Learn Data Science?
The answer to this question depends on many factors, such as the way of learning you choose (book-based or video-based self-tuition, in a school, a boot camp, a master’s program, etc.), the curriculum you follow, how many hours you are ready to dedicate to learn data science, your initial background, etc. On average, to a person with no prior coding experience and/or mathematical background, it takes from 7 to 12 months of intensive studies to become an entry-level data scientist.
It is important to keep in mind that learning only the theoretical basis of data science may not make you a real data scientist. Whatever program you choose, you should pay attention to practicing your skills, making data science projects, creating your project portfolio, exploring data science use cases in various spheres, and experimenting with alternative approaches to solving the same data science task. All these activities, if conducted with diligence and persistence, can be rather time-consuming. However, this is the best way to master your data science skills and gain job-ready proficiency.
To accelerate your learning process, consider opting for an online self-study program with a well-balanced curriculum that covers the most important techniques and aspects of data science. This will help you efficiently manage your time, decide on the most comfortable and productive approach to learning the materials, and allow you to learn at your own pace from wherever you have a computer and Internet access. With Chools, you can select from fully-packed Career tracks for very beginners, specialized Skill Tracks to sharpen particular skills, and short courses to explore narrow-focused topics.
How Proficient Should a Data Scientist Be in Coding?
While coding is an essential skill for any data science job, expertise in programming is not mandatory to get started in this sphere. No doubt, a person who wants to land a job in data science should be familiar with certain programming languages and related technical tools, and the companies that hire data scientists usually require such skills. However, the coding toolkit of a data scientist is definitely not as extensive as that of, say, a software developer or a computer scientist. The choice of programming languages relevant to solving data science tasks is also quite limited, and learning the basic data-related methods and techniques of only one of them can be a great place to start.
Rather than being a purely programming-focused discipline, data science is a vast field of study that requires a diverse set of skills and competencies apart from coding, such as having an analytical mindset, understanding statistics, probability, linear algebra, efficient storytelling, and business domain knowledge.
What Are the Most Important Programming Languages to Learn to Become a Data Scientist?
There are three programming languages that are widely used in data science: Python, R, and SQL.
Python is an open-source, object-oriented, high-level programming language that was originally used for general-purpose programming in computer science, but later became very popular in data science. Its main advantages are an extensive standard library and a big collection of additional modules which are particularly helpful for solving data science tasks. In addition, Python is intuitively understandable and easy to learn and use, can be run in many operating systems, and is supported by a strong community.
R is a popular data science-oriented programming language and free software that is very powerful in statistical computing and data visualization. Just like Python, it provides many data science and machine learning libraries for solving different tasks, is operating system friendly, and has excellent online support. However, this programming language is considered to be less intuitive than Python.
While Python and R are mostly similar in their functionality, SQL (Structured Query Language) is used for a different purpose: to query relational database management systems such as tables with connected data entries. SQL has several flavors, all with a rather similar syntax; some of them are free and open source (e.g., MySQL, SQLite, PostgreSQL).
What Mathematical Background is Required of a Data Scientist?
First of all, you don’t need any mathematical background to start learning data science. On the other hand, if you have decided to become a data scientist and are ready to make efforts for it, you will inevitably have to get familiar with some mathematical concepts related to data science. Apart from the very basics of math taught in a common school program, you will need knowledge of calculus, probability, statistics, and linear algebra.
However, this doesn’t mean that you must learn the above subjects from beginning to end. Moreover, the majority of math is already included in data science tools and methods, so many complex operations are just calculated by the machine under the hood based on the input parameters. A data scientist is, above all, a scientist, so he or she does have to understand how and why all those algorithms work behind the scenes to be able to select the best one, define the initial parameters, and adjust them properly. In Chools Skills tracks, Career tracks, career tracks and courses, you will be introduced on a step-by-step basis to all the necessary theory in mathematics that is applicable to solve various real-world tasks in data science.
Where Should I Look for a Data Science Job?
The first place that comes to mind is free job listing websites. You can consider using both general job portals etc. (LinkedIn, Indeed, Google for Jobs, SimplyHired, AngelList, Hired,) and data science niche job boards (KDNuggets, DataJobs, Amazon Jobs, StatsJobs, etc.). There are also websites designed for searching for remote jobs : Upwork, Remote, JustRemote, We Work Remotely. You could also use specialized job boards, such as Outer Join that are dedicated to remote jobs exclusively in the data science sphere.
Apart from that, you can try to reach out to a company of interest directly. Find their official website, explore its home page, career page, and contact details. Read their values and mission, what their business looks like, and consider how you could be a perfect fit for this organization. Armed with this information, you can send them an email with your data science resume attached. This approach, while potentially more time-consuming, is more advantageous than the first one since it allows you to show a genuine interest in the company and stand out from the crowd.
To have more chance of finding a data science job quickly, it can be helpful to attend data science events and conferences (both live and online), connect with the right people on social networks, and communicate with data science professionals and learners in specialized data science communities. At Chools, you will find a friendly community of data science enthusiasts where you can get help and support and expand contacts in the world of data science.
What Skills and Qualities Do Employers Look for in a Data Scientist?
The most basic technical skills that employers usually expect from a data scientist include:
- good command of Python or R (especially the popular data science modules of these languages)
- competence in SQL
- the ability to work with the command line
- understanding of statistical concepts,
- data cleaning, wrangling, analysis, and visualization skills
- predictive modeling and model estimation using machine learning or deep learning algorithms
- working with unstructured data
- storytelling
- web scraping
- debugging
This doesn’t mean that you would necessarily need all those skills for any data science position. To understand what each particular company wants to see in a data scientist, you should read the corresponding job description and make a list of the specific technical skills and tools they require.
As for the necessary soft skills for a data scientist, the most sought-after ones are:
- critical thinking
- team working
- business domain knowledge
- efficient communication
- decision making
- multitasking
- flexibility
- curiosity
- creativity
- ability to meet tight deadlines
What Should I Keep in Mind while Searching for a Data Science Job?
The first thing is to have a prepared portfolio of projects. This is especially important for those candidates who lack real working experience in this sphere. Such a portfolio should include the projects that you completed as a part of your data science bootcamp or course. In addition, consider making 2-3 more projects that will make your portfolio unique. For an entry-level data scientist or a career-changer, it is perfectly ok if at the beginning your portfolio contains projects on mixed topics and techniques. However, when applying to a particular job position, try to figure out which of your works highlights the best of all your skills required for that job.
The next crucial point is your data science resume. Before applying for different job positions, consider creating a master version of your resume where you put all the information about your education, working experience (even if not data-related), courses, boot camps, projects, technical and soft skills, and any other achievements that could be relevant in any way. Don’t worry if this version of your resume is rather long, or is made up of multiple sections and subsections. Now, whenever you want to apply for a particular data science job, you can just use your master resume as a basis. Simply create a copy of it, remove all the redundant details and sections, and tailor your resume for that specific position according to the job description. Remember that adapting your resume for each submission is a necessary step in your job search process. If you need more tips on how to create an excellent, professional-looking data science resume, you can find this article helpful.
The third essential thing that you should be aware of is that you might not manage to find a data science job immediately. If this happens, don’t be discouraged. It is absolutely normal if your job search process takes time. Don’t let eventual rejections frustrate you and make you start thinking that you are not a good specialist. Instead, continue mastering your data science skills and try to analyze what can be adjusted in your resume, your portfolio of projects, and the application process in general. If you get some feedback from any of the companies you apply to, make the most of this information by improving on any of your highlighted weaknesses.
Our Data Science courses with new-age curriculum
Acquire the most in-demand skills to propel your career in Data Science
# Learn the distribution and real-time analysis of data by applying Statistics.
# Perform Statistical analysis, data visualization, and predection using Python
# Tackle Big Data with PySpark using distributed computing.
# Use SQL to manipulate databases.
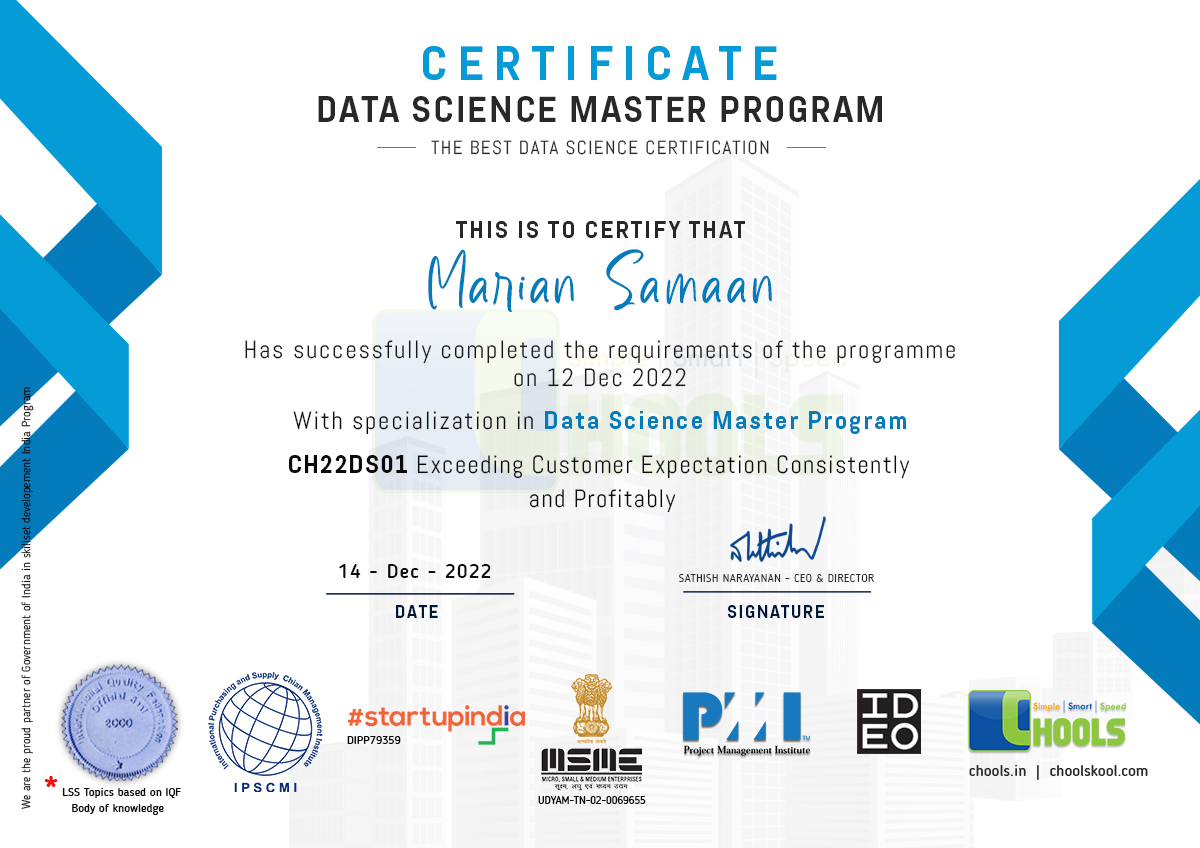
Our Data Science Leaders panel
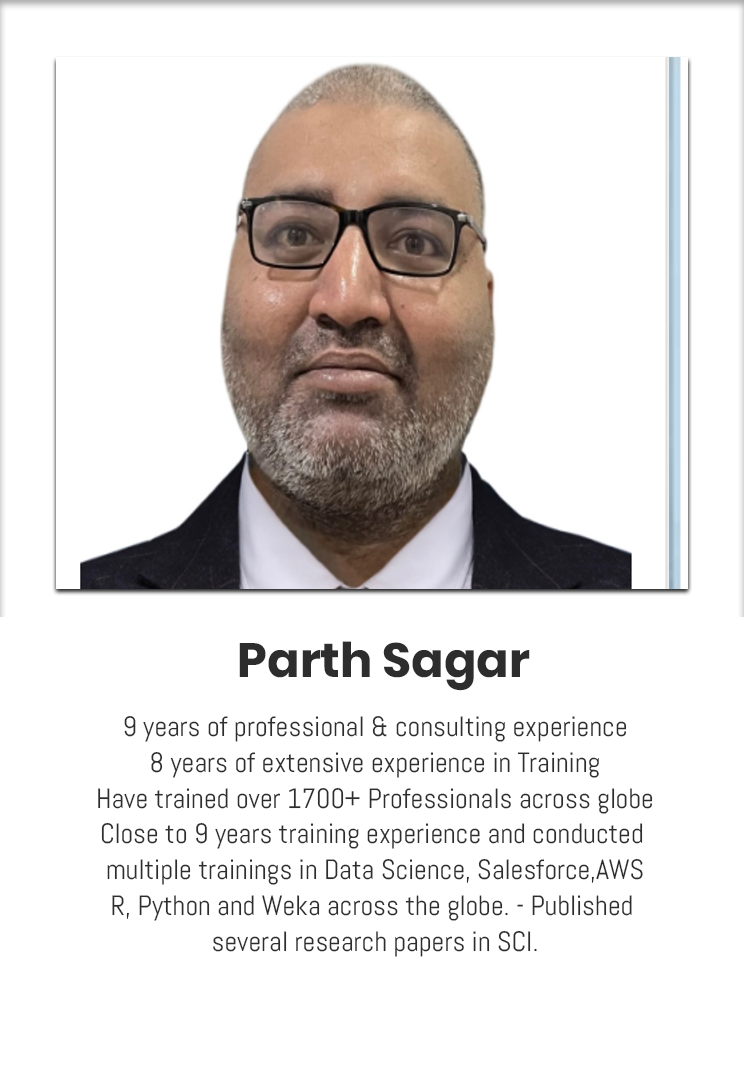
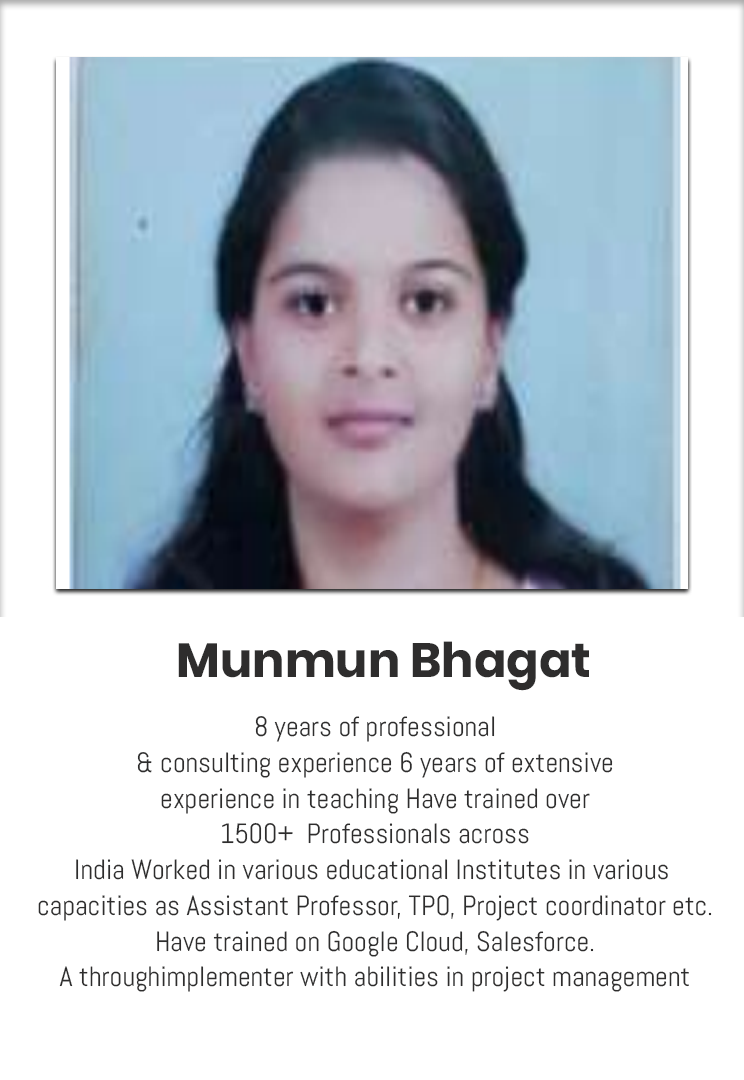
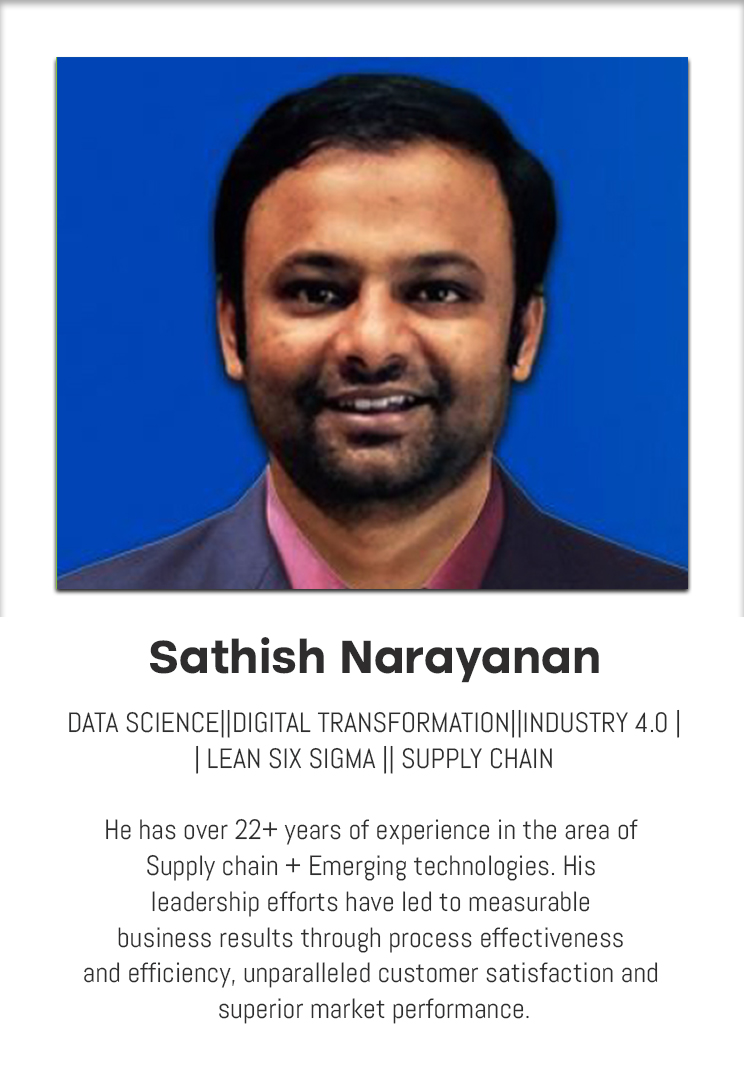
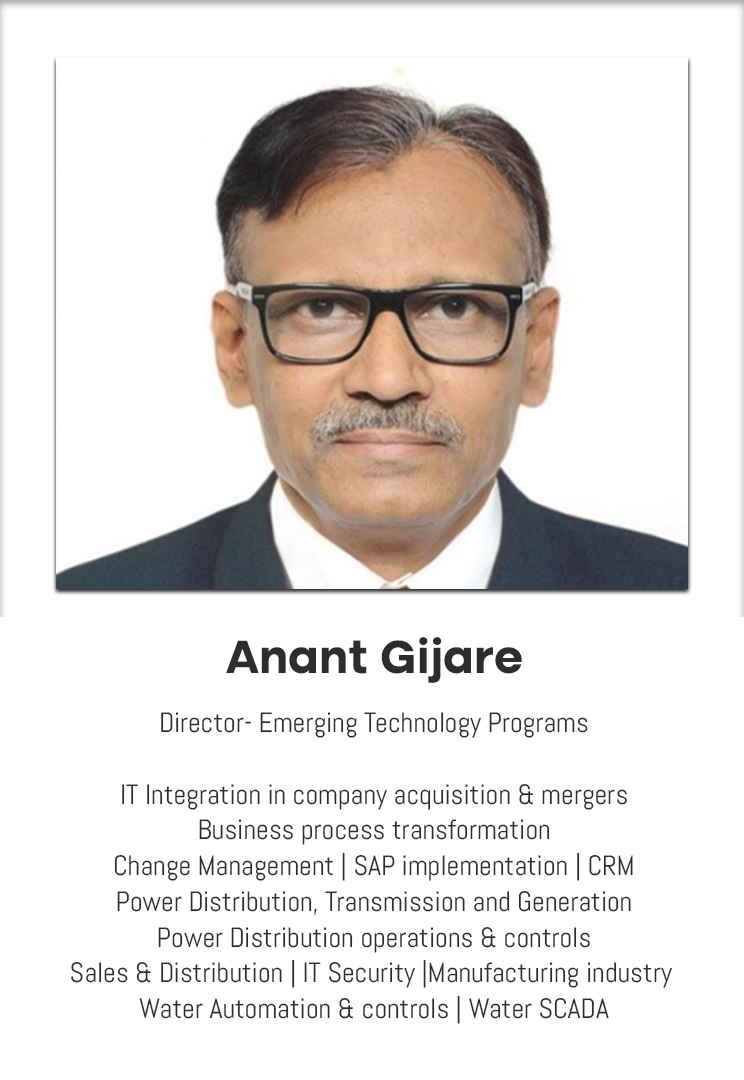
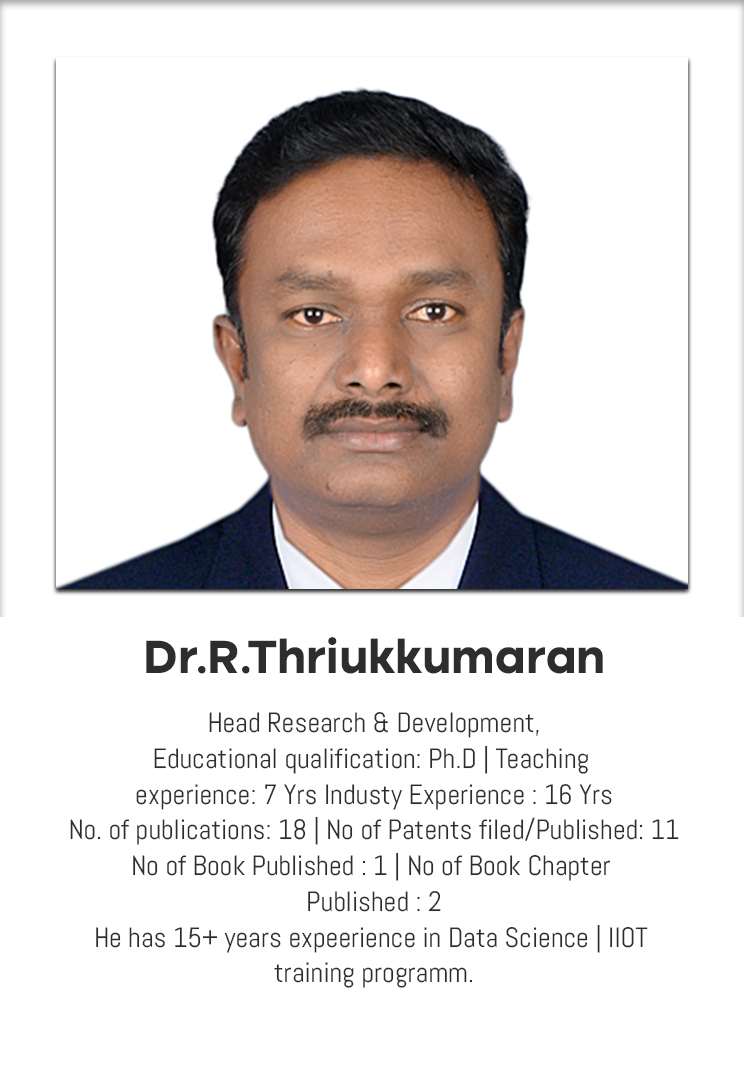
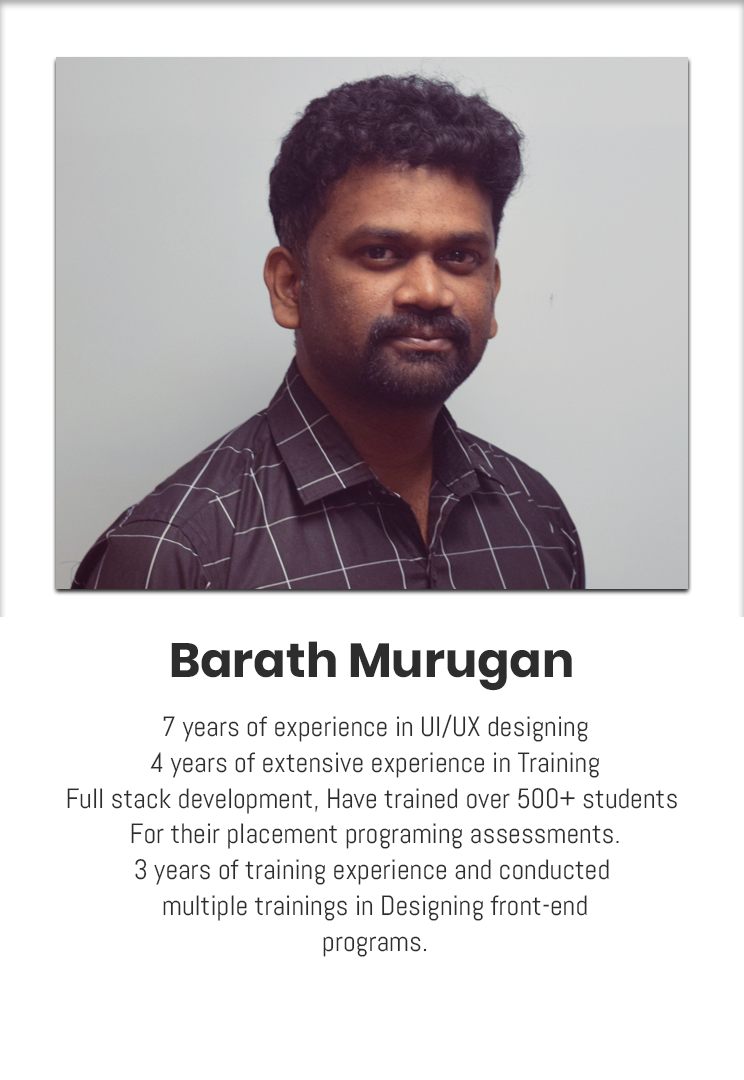
Frequently Asked Questions on Machine Learning & AI
What is the difference between machine learning and deep learning?
Machine learning or ML is a subset of Artificial intelligence. The core objective of this field is to create algorithms that will set some variable so that the machine can think for itself when they encounter those variable in other data sets. Deep learning or DL, on the other hand, is more complex as the programming aims to mimic how the human brain operates. The deep learning algorithms have artificial neural systems that function as the human neural system. Deep learning is more suitable for complex and very large data sets.
What is an algorithm?
An algorithm is a set of instructions that are set finitely. In order to explain this better, imagine the set of instructions that are provided with a recipe. There are ingredients and there are set steps that are provided for cooking that specific dish. Algorithms are the same set of instructions for a computer or mathematical program. Some commonly used algorithms are:
Naïve Bayes Classifier Algorithm
- K-Means Clustering Algorithm
- Support Vector Machine Algorithm
- Linear Regression
- Logistic Regression
- Artificial Neural Networks
What is the job of a machine learning engineer?
A machine learning engineer has a job description that is similar to that of a data scientist or any other data science course career path. But, a machine learning engineer is more focused on the creation of machine learning algorithms. These algorithms could work with little to no supervision from humans.
What is the career path after completing a course in machine learning?
Like any other data-related field, machine learning also provides a widely diverse career path. While the machine learning engineer is the primary career option, you can even become a data scientist or a data analyst after completing the course. The more specialization you have, the more complex your job will get and that will also positively impact your earnings.
How are artificial intelligence machine learning and data science related?
In simple terms, artificial intelligence is the umbrella term. It is the final product that we see and use. Our Ai-powered devices are an example of artificial intelligence that constantly evolves to make smarter decisions. Backing up this artificial intelligence are machine learning and data science. Data science discerns the patterns that the user is generating and machine learning updates the program to work on the predicted outcomes.
What is meant by semi-supervised algorithms?
If you know about the working of the anomaly detection program, you will have an understanding of semi-supervised algorithms. This type of algorithm is made to understand a specific set of problems and then that understanding is made to be implied on a larger scale.
How can you become a machine learning engineer without having a computer science background?
Technically, you cannot become a machine learning engineer if you have no inkling about the basic concepts of computer science and programming languages. If you did not have computers as a subject in school, you can opt for crash courses to accelerate your learning. This will also help you get into any renowned institute as it depicts your learning enthusiasm.
What programming languages are needed in machine learning?
Machine learning requires a basic understanding of the web, the data sets, and the coding languages. The best languages for these purposes are:
- Python
- Java
- C++
- R
- JavaScript
This is another fundamental difference between data science and machine learning. In data science, Javascript is the preferred language while in machine learning it is Python.
Which Python libraries are preferred by machine learning scientists and students?
Being a widely-used programming language for machine learning, Python has various libraries that make the work of a machine learning engineer easier. These libraries are:
Numpy: Numeric Python or Numpy is a Linear Algebra Library for Python with powerful data structures for efficient computation of multi-dimensional arrays and matrices.
- Pandas is the most popular Python library known for providing optimized performance for data analysis.
- Matplotlib is a popular python plotting library that creates basic graphs like line charts, bar charts, or histograms.
- Seaborn is perfect for generating attractive graphs.
What is the function of machinelearn.js?
Even though Python serves to be the major programming language for machine learning, it is Javascript’s machinelearn.js that helps the algorithm to be able to provide features like decomposition or clustering to the models. This also helps with supervised and unsupervised machine learning.
How is machine learning related to Big Data?
Just like the spinal cord is the backbone of the whole human body, Big Data is mainly supported by machine learning. Big Data is a set of complex and unstructured data. But, even though there is a great amount to search through, the benefit of Big Data is that you can have many aspects related to a specific data set. Machine learning makes handling and streamlining Big Data easy.
Can you do a Ph.D. in machine learning?
Yes, like any other discipline, it is possible to obtain a Ph.D. degree in machine learning. This is done after completing a master’s degree and after successfully passing the NET test that is required for all doctorate aspirants.
What is the job of a computational linguist?
Another career option after completing a machine learning course is a computational linguist is responsible for assisting Ai-enabled speech recognition synthesis. The job description for a computational linguist is:
Building applications for integrating human language
- Maintaining track of online programs and search engines
- Communicating with other experts in this field to understand and incorporate new findings
- Integrating newer languages
Can you learn machine learning on your own?
There are many platforms and machine learning tutorials available online, and for free. But, these are not suitable for freshers who have just begun understanding the basics of machine learning. Self-study is possible only when you have a good understanding of data science, artificial intelligence, and coding along with programming and generating predictive models.
Hence, it is advised that if you have just graduated from school, you can opt for an online course first to understand the basics. These include Bootcamp courses. This will help you also decide if machine learning is for you.
Can machine learning be learned for free?
Some courses are curated by developers and machine learning experts that are offered for free and are perfect for beginners looking to understand if machine learning is their cup of tea or not. Some of these are:
- Machine Learning By Andrew Ng
- Deep Learning and NumPy stack
- Practical Machine Learning with Scikit-Learn
What is the curriculum for the master’s degree in machine learning?
At the master’s level, there are six core subjects that the students take. These courses are:
- Introduction to Machine Learning or Advanced Introduction to Machine Learning
- Intermediate Deep Learning or Deep Reinforcement Learning or Advanced Deep Learning
- Probability & Mathematical Statistics
- Convex Optimization
- Probabilistic Graphical Models
- Data Analysis or Machine Learning in Practice
Apart from these, there are various electives that the students can opt for. These electives are:
- Machine Learning with Large Datasets
- Advanced Deep Learning
- Statistical Machine Learning
- Neural Networks for NLP
- Graduate Artificial Intelligence
- Regression Analysis
What is the programming language R?
The R programming language is ideal for statistically driven libraries that enable machine learning to make predictive analysis more effective. While Python is known to be the most suitable programming language for machine learning, it is ideal for beginners and even experts who are only working with the basics. With R, the people who work with data experimentation and exploration find it easy.
What is the main difference between statistical modeling and machine learning?
The primary difference lies in the approach that is taken by both these fields. A statistical modeling approach is more focused on implying parameters, like logistic regression or linear regression. On the other hand, machine learning is more about nonparametric approaches like nearest trees or kernel SVM.
Which machine learning algorithms can be considered to be the best?
As machine learning is primarily about algorithms, there need to be some preferred algorithms that the machine learning field prefers over others. As a broader classification, the machine learning algorithm is divided into three parts: Supervised learning, Unsupervised learning, and Reinforcement learning.
The most preferred learning algorithms are:
- Linear regression
- Logistic regression
- Decision tree
- SVM algorithm
- Naive Bayes algorithm
- KNN algorithm
- K-means
What is Natural Language Processing?
A subfield of linguistics, computer science, and artificial intelligence, Natural Language Processing helps facilitate the communication between humans and computers. This is primarily used to help a computer process a large amount of data related to the natural language.