6 Months
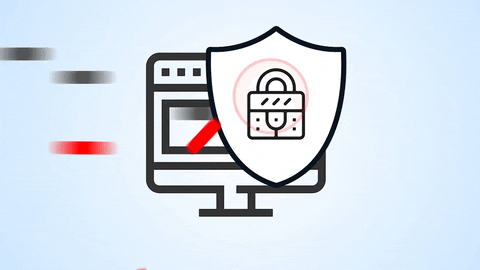
- Statistics
- Data visualization in python
- EDA
- Regression
- Supervised Machine Learning
- Unsupervised Machine Learning
- Ensemble Techniques
- Association Rule
- Recommendation system
- Artificial Neural Network
- CNN
- Setting up a malware analysis environment
- Performing static and dynamic malware analysis
- Building an intrusion detection system
- Using machine learning for social engineering
- Enriching pretesting via machine learning
- Machine Learning for Intrusion Detection
- Malware Detection via Machine Learning
- Preparation for Cybersecurity Data Science
- Writing scripts to efficiently read and manipulate CSV, XML, and JSON files
- Quickly and efficiently parsing executables, log files, pcap and extracting artifacts from them
- Making API calls to merge datasets
- Use the Pandas library to quickly manipulate tabular data
- Effectively visualizing data using Python
- Preprocessing raw security data for machine learning and feature engineering
- Building, applying and evaluating machine learning algorithms to identify potential threats
- Automating the process of tuning and optimizing machine learning models
- Hunting anomalous indicators of compromise and reducing false positives
- Use supervised learning algorithms such as Random Forests, Naive Bayes, K-Nearest Neighbors (K-NN) and Support Vector Machines (SVM) to classify malicious URLs and identify SQL Injection
- Apply unsupervised learning algorithms such as K-Means Clustering to detect anomalous behavior
- Assignments for assessment
- Projects
Internship
Course Outline
Statistical Foundations
In this module, you will learn everything you need to know about all the statistical methods used for decision making in this Data Science course.
- Probability distribution – Binomial, Poisson, and Normal Distribution in Python.
- Bayes’ theorem – Baye’s Theorem is a mathematical formula named after Thomas Bayes, which determines conditional probability. Conditional Probability is the probability of an outcome occurring predicated on the previously occurred outcome.
- Central limit theorem – This module will teach you how to estimate a normal distribution using the Central Limit Theorem (CLT).
- Hypothesis testing – This module will teach you about Hypothesis Testing in Statistics. One Sample T-Test, Anova and Chi-Square test.
Exploratory Data Analysis (EDA)
This module of 6 months in Data Science courses will teach you all about Exploratory Data Analysis like Pandas, Seaborn, Matplotlib, and Summary Statistics.
- Pandas – Pandas is one of the most widely used Python libraries. Pandas is used to analyze and manipulate data. This module will give you a deep understanding of exploring data sets using Pandas.
- Summary statistics (mean, median, mode, variance, standard deviation) – In this module, you will learn about various statistical formulas and implement them using Python.
- Seaborn – Seaborn is also one of the most widely used Python libraries. Seaborn is a Matplotlib based data visualization library in Python. This module will give you a deep understanding of exploring data sets using Seaborn.
- Matplotlib – Matplotlib is another widely used Python library. Matplotlib is a library to create statically animated, interactive visualizations. This module will give you a deep understanding of exploring data sets using Matplotlib.
Regression- Linear Regression
This module will get us comfortable with all the techniques used in Linear and Logistic Regression.
- Multiple linear regression – Multiple Linear Regression is used for predicting one dependent variable using various independent variables.
- Fitted regression lines – A fitted regression line is a mathematical regression equation on a graph for your data.
- AIC, BIC, Model Fitting, Training and Test Data – In this module, you will go through everything you need to know about several models such as AIC, BIC, Model Fitting, Training, and Test Data.
Regression- Logistic Regression
- Introduction to Logistic regression, interpretation, odds ratio – It is a simple classification algorithm to predict the categorical dependent variables with the assistance of independent variables.
- Misclassification, Probability, AUC, R-Square – This module will teach everyone how to work with Misclassification, Probability, AUC, and R-Square.
Supervised Machine Learning
In the next module, you will learn all the Supervised Learning techniques used in Machine Learning.
- CART – CART is a predictive machine learning model that describes the prediction of outcome variable’s values predicated on other values.
- KNN – KNN is one of the most straightforward machine learning algorithms for solving regression and classification problems.
- Decision Trees – Decision Tree is a Supervised Machine Learning algorithm used for both classification and regression problems. It is a hierarchical structure where internal nodes indicate the dataset features, branches represent the decision rules, and each leaf node indicates the result.
- Naive Bayes – Naive Bayes Algorithm is used to solve classification problems using Baye’s Theorem.
Unsupervised Learning
In the next module, you will learn all the Unsupervised Learning techniques used in Machine Learning.
- Clustering – K-Means & Hierarchical – Clustering is an unsupervised learning technique involving the grouping of data. In this module, you will learn everything you need to know about the method and its types, like K-means clustering and hierarchical clustering.
- Distance methods – This module will teach you how to work with all the distance methods or measures such as Euclidean, Manhattan, Cosine.
- Features of a Cluster – Labels, Centroids, Inertia – This module will drive you through all the features of a Cluster like Labels, Centroids, and Inertia.
- Eigen vectors and Eigen values – In this module, you will learn how to implement Eigenvectors and Eigenvalues in a matrix.
- Principal component analysis – Principal Component Analysis is a technique to reduce the complexity of a model, like eliminating the number of input variables for a predictive model to avoid overfitting.
Ensemble Techniques
In this Machine Learning, we discuss supervised standalone models’ shortcomings and learn a few techniques, such as Ensemble techniques, to overcome these shortcomings.
- Bagging & Boosting – Bagging is a meta-algorithm in machine learning used for enhancing the stability and accuracy of machine learning algorithms, which are used in statistical classification and regression.
Boosting is a meta-algorithm in machine learning that converts robust classifiers from several weak classifiers. - Random Forest – Random Forest comprises several decision trees on the provided dataset’s several subsets. Then, it calculates the average for enhancing the dataset’s predictive accuracy.
- AdaBoost & Gradient boosting – Boosting can be further classified as Gradient boosting and ADA boosting or Adaptive boosting. This module will teach you about Gradient boosting and ADA boosting.
Association Rules Mining & Recommendation Systems
Association rule mining is the data mining process of finding the rules that may govern associations and causal objects between sets of items.
Recommendation engines are a subclass of machine learning which generally deal with ranking or rating products / users. Loosely defined, a recommender system is a system which predicts ratings a user might give to a specific item. These predictions will then be ranked and returned back to the user.
- Understanding to Deep Learning – Single Layer Perceptron
Artificial neural networks, usually simply called neural networks or neural nets, are computing systems inspired by the biological neural networks that constitute animal brains. An ANN is based on a collection of connected units or nodes called artificial neurons, which loosely model the neurons in a biological brain.
- Convolutional Neural Network
A convolutional neural network is a feed-forward neural network that is generally used to analyze visual images by processing data with grid-like topology. It’s also known as a ConvNet. A convolutional neural network is used to detect and classify objects in an image.
- Setting up a malware analysis environment
- Performing static and dynamic malware analysis
- Building an intrusion detection system
- Using machine learning for social engineering
- Enriching pretesting via machine learning
- Machine Learning for Intrusion Detection
- Malware Detection via Machine Learning
- Preparation for Cybersecurity Data Science
- Writing scripts to efficiently read and manipulate CSV, XML, and JSON files
- Quickly and efficiently parsing executables, log files, pcap and extracting artifacts from them
- Making API calls to merge datasets
- Use the Pandas library to quickly manipulate tabular data
- Effectively visualizing data using Python
- Preprocessing raw security data for machine learning and feature engineering
- Building, applying and evaluating machine learning algorithms to identify potential threats
- Automating the process of tuning and optimizing machine learning models
- Hunting anomalous indicators of compromise and reducing false positives
- Use supervised learning algorithms such as Random Forests, Naive Bayes, K-Nearest Neighbors (K-NN) and Support Vector Machines (SVM) to classify malicious URLs and identify SQL Injection
- Apply unsupervised learning algorithms such as K-Means Clustering to detect anomalous behavior
- Assignments for assessment
- Projects
- Internship