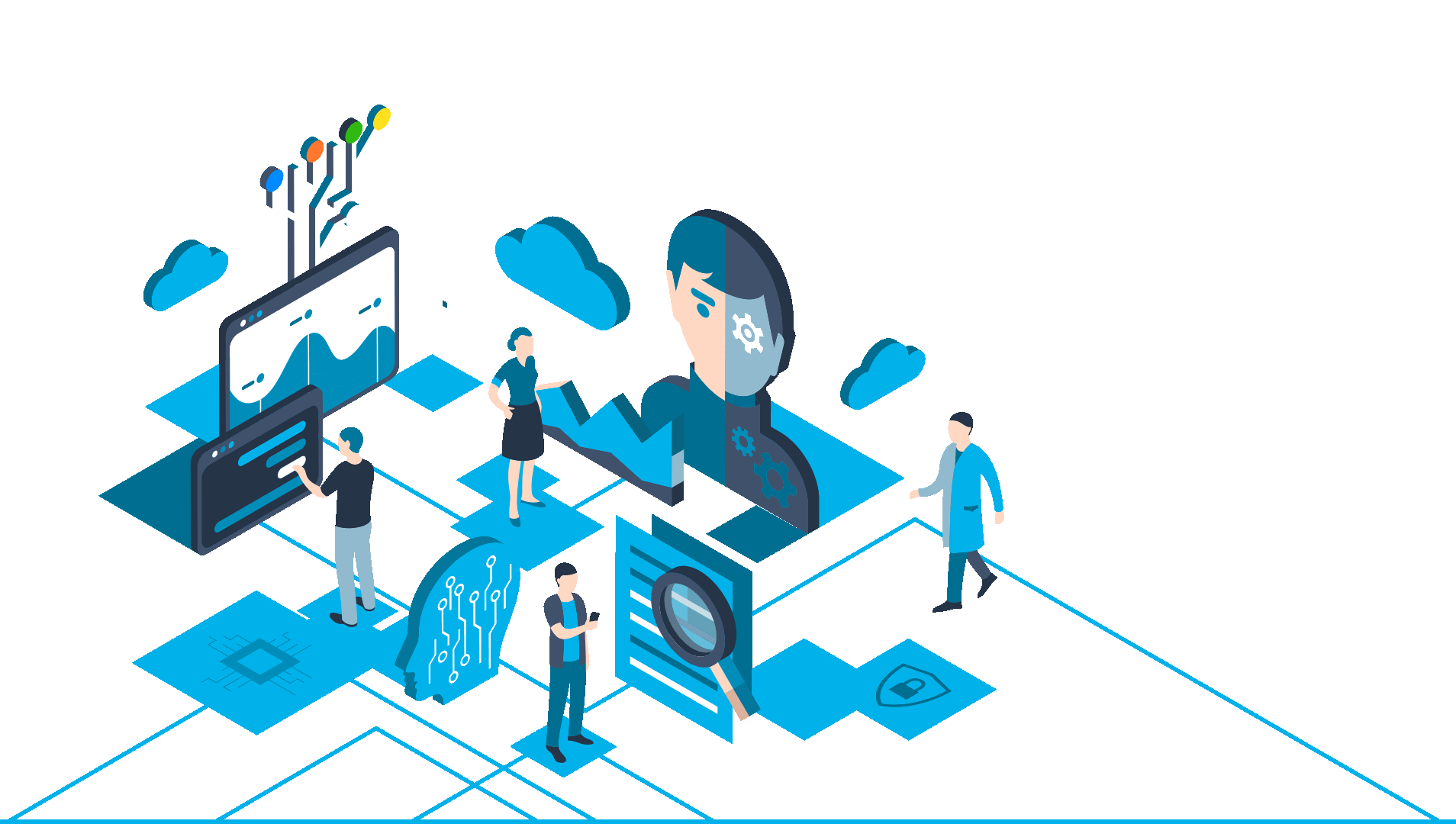
Introduction
It’s the age of machine learning. Companies are seizing upon the power of this technology to combat risk, boost sales, cut costs, block fraud, streamline manufacturing, conquer spam, toughen crime fighting, and win elections.
This course will prepare you to participate in the deployment of machine learning – whether you’ll do so in the role of enterprise leader or quant. In order to serve both types, this course goes further than typical machine learning courses, which cover only the technical foundations and core quantitative techniques. This course includes illuminating software demos of machine learning in action using SAS products. However, the curriculum is vendor-neutral and universally-applicable. The contents and learning objectives apply, regardless of which machine learning software tools you end up choosing to work with.
What You’ll Learn
- Participate in the deployment of machine learning
- Identify potential machine learning deployments that will generate value for your organization
- Report on the predictive performance of machine learning and the profit it generates
- Understand the potential of machine learning and avoid the false promises of “artificial intelligence”
What you will learn from this course
Module 1 – Introduction
➤ Machine learning specialization overview
➤ Why this course and what you will learn
➤ Vendor-neutral courses
➤ Exploring SAS, Visual Data Mining, and Machine Learning
Module 2 – The impact of Machine Learning
➤ Forecasting vs. predictive analytics
➤ The full definitions of machine learning and predictive
analytics
➤ The two stages of machine learning: modeling and
scoring
➤ Targeting marketing with response modeling
➤ The Prediction effect
➤ Targeted customer retention with churn modelling
➤ Financial credit risk
➤ “Non-predictive” applications: detection and Classification
➤ Why ML is the latest evolutionary step of the Information Age
Module 3 – Data
➤ Introduction to Big Data
➤ A paradigm shift for scientific
discovery: its automation
➤ The Data Effect: Data is always predictive
➤ Training data — what it looks like
➤ Predicting with one single variable
➤ Growing a decision tree to combine variables
➤ More on decision trees
➤ The light bulb puzzle
➤ Measuring predictive performance
➤ DEMO – Training a simple decision tree model (optional)
Module 4 – Predictive Models
➤ The principles of predictive modelling
➤ How can you trust a predictive model
➤ More predictive modeling principles
➤ Visually comparing modeling methods
– decision boundaries
➤ DEMO – Training and comparing multiple models
➤ Deploying a predictive model
➤ The profit curve of a model
➤ Deployment results in targeting marketing and sales
➤ Deep learning – application areas and limitations
➤ Labeled data: a source of great power, yet a major limitation
➤ Talking computers — natural language processing and text analytics
Module 5 – Industry Perspective: AI Myths and Real Ethical Risks
➤ Dismantling the logical fallacy that is AI
➤ Why legitimizing AI as a field incurs great cost
➤ Ethics overview: five ways ML threatens social justice
➤ Blatantly discriminatory models
➤ The trend towards discriminatory models
➤ The argument against discriminatory models
➤ Five myths about “evil” big data
➤ Defending machine learning — how it does good
➤ Course wrap-up